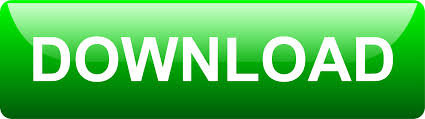
Researchers therefore have applied off-policy correction on the learning of recommender systems to address such biases. However, such data are inherently biased because the feedback can only be observed on items recommended by the historical systems. Fortunately, abundant logged user feedback (e.g., user clicks or dwell time) generated by historical recommender systems is available and commonly used as training data. To achieve this goal, a two-stage approach is widely used, where an efficient candidate generation model generates a candidate set of hundreds of items from the whole item space at the first stage, and then, at the second stage, a more powerful ranking model re-ranks the candidate items and recommends the top few items to the user.Another major challenge is how to get enough labeled data to train such large-scale recommender systems. The large scale and the strict latency have led to numerous technical challenges.One major challenge is how to serve the users efficiently with highly personalized content. And moreover, the systems are required to respond users' request in real time within milliseconds. Chi (Google).ĪbstractRecommender systems in industrial production often need to serve billions of users with a million-level candidate item space to recommend from.
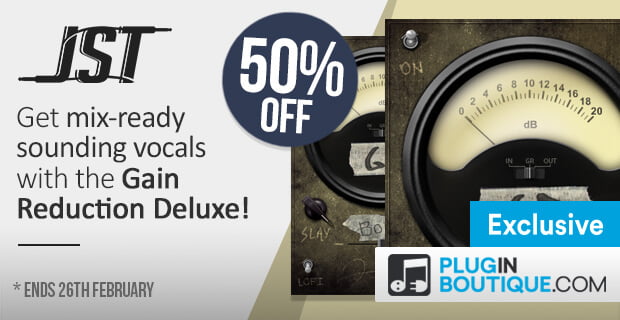
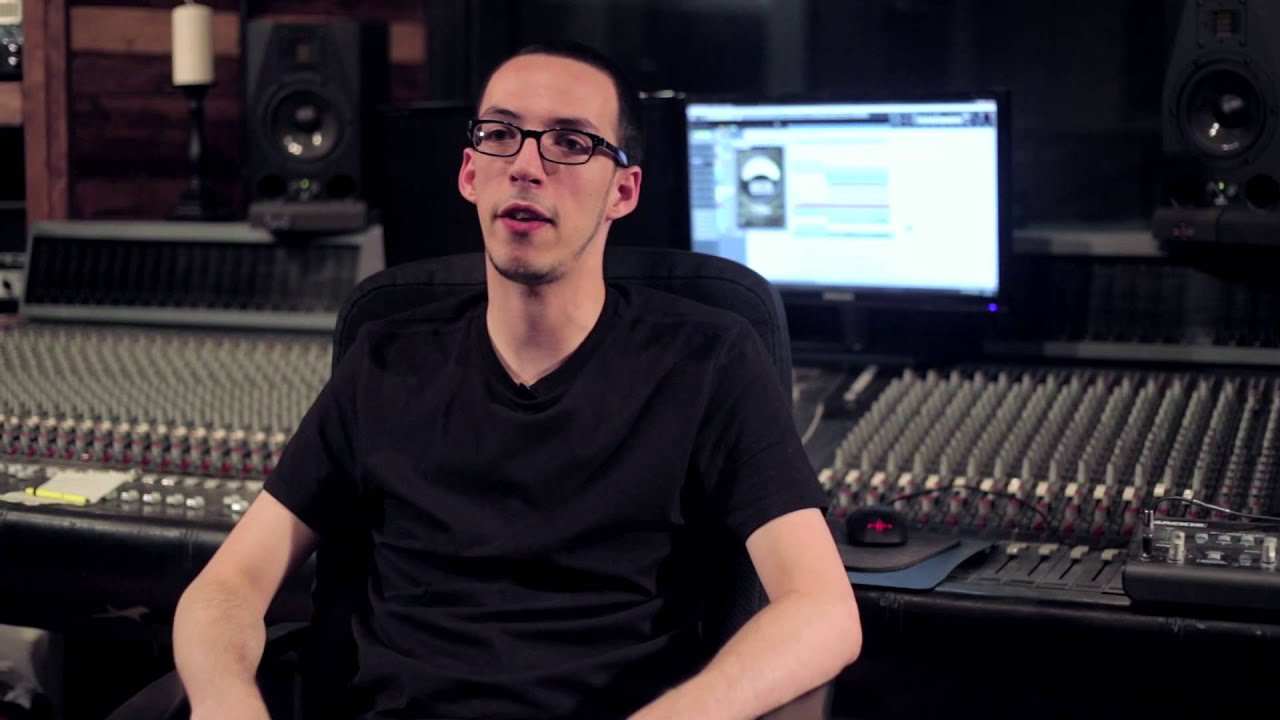
Jiaqi Ma (University of Michigan), Zhe Zhao (Google), Xinyang Yi (Google), Ji Yang (Google), Minmin Chen (Google), Jiaxi Tang (Simon Fraser University), Lichan Hong (Google) and Ed H. Off-policy Learning in Two-stage Recommender Systems
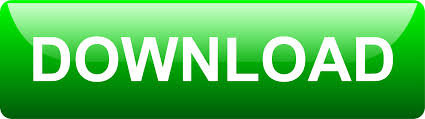